
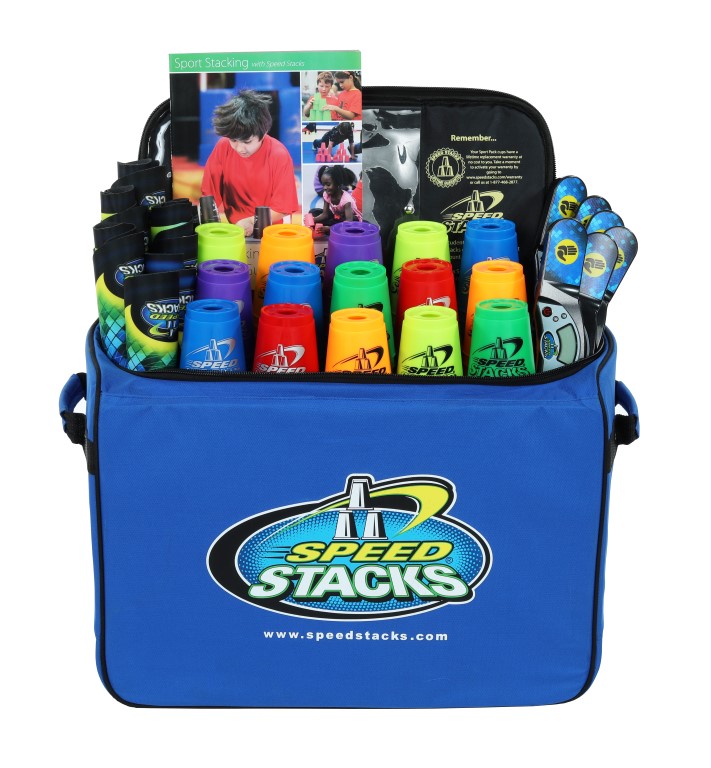
CPU is a sports car, GPU is a massive truckīecause of the CPU limited throughput (indeed CPU as around a 10x slower throughput than GPUs).
STACK SPORTS PCI FULL
This is resulting in a non controlled usage of the GPU by the Hypervisor meaning that you are, as a customer, accessing the RAW hardware at full performances. The hypervisor is therefore giving to the virtual Machine a plain access to the GPU through the PCI-e BUS. PCI Express pass-through concept is that the KVM will not emulate the GPU address but will pass the instruction directly to the Graphical Processing Unit to the physical address of the device. At each CPU cycle the kernel will potentially move the Virtual Machine from the attached CPU to another CPU and this is when things can get dirty. The virtualization at the KVM level will attribute a CPU time for each VM using CPU cycles. It was important to define how virtualization works to understand the limitations. Why PCI Express pass-through and associated limitations ? Now let’s talk about the CPUs/GPUs virtualization trap. Here are 2 representations of a AI virtualized and dockerized environment. This mode provides slightly better performance (between 1 and 2 percents).
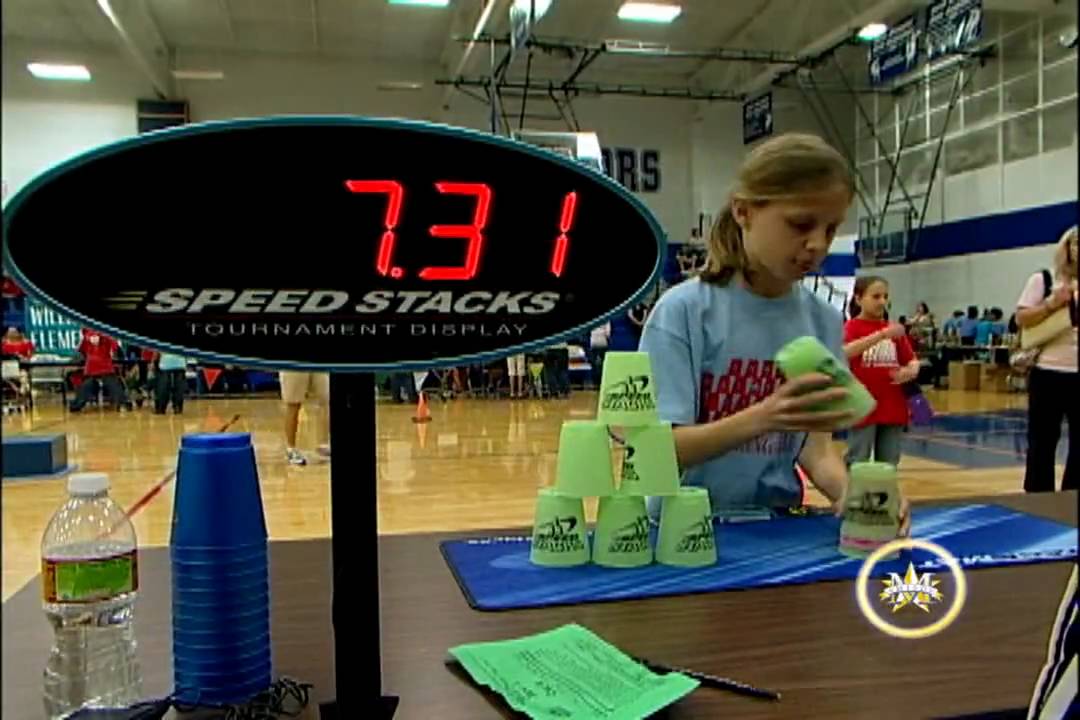
In this case we will use VFIO that will assign the physical address of the GPU to the guest VM. passthrough-ization (I know… this word doesn’t exits …) where you have direct access to the physical hardware.Multiple market solution exist (citrix, vmware, NVIDIA GRID for VDI, NVIDIA Quadro Virtual Data Center Workstation, NVIDIA Virtual Compute Server (vCS), …) In this case the GPU is accessed through a virtualized material address and not directly. virtualization of the GPU ( vGPU) or a slice of it where you give access to a slice of instruction to the GPU with an intermediate interpreter.emulation through nvemulate (Nvidia) or OpenCL device emulator (AMD) where you emulate instruction (cuda or opencl).There are multiple ways to handle GPUs in a virtualized environment:
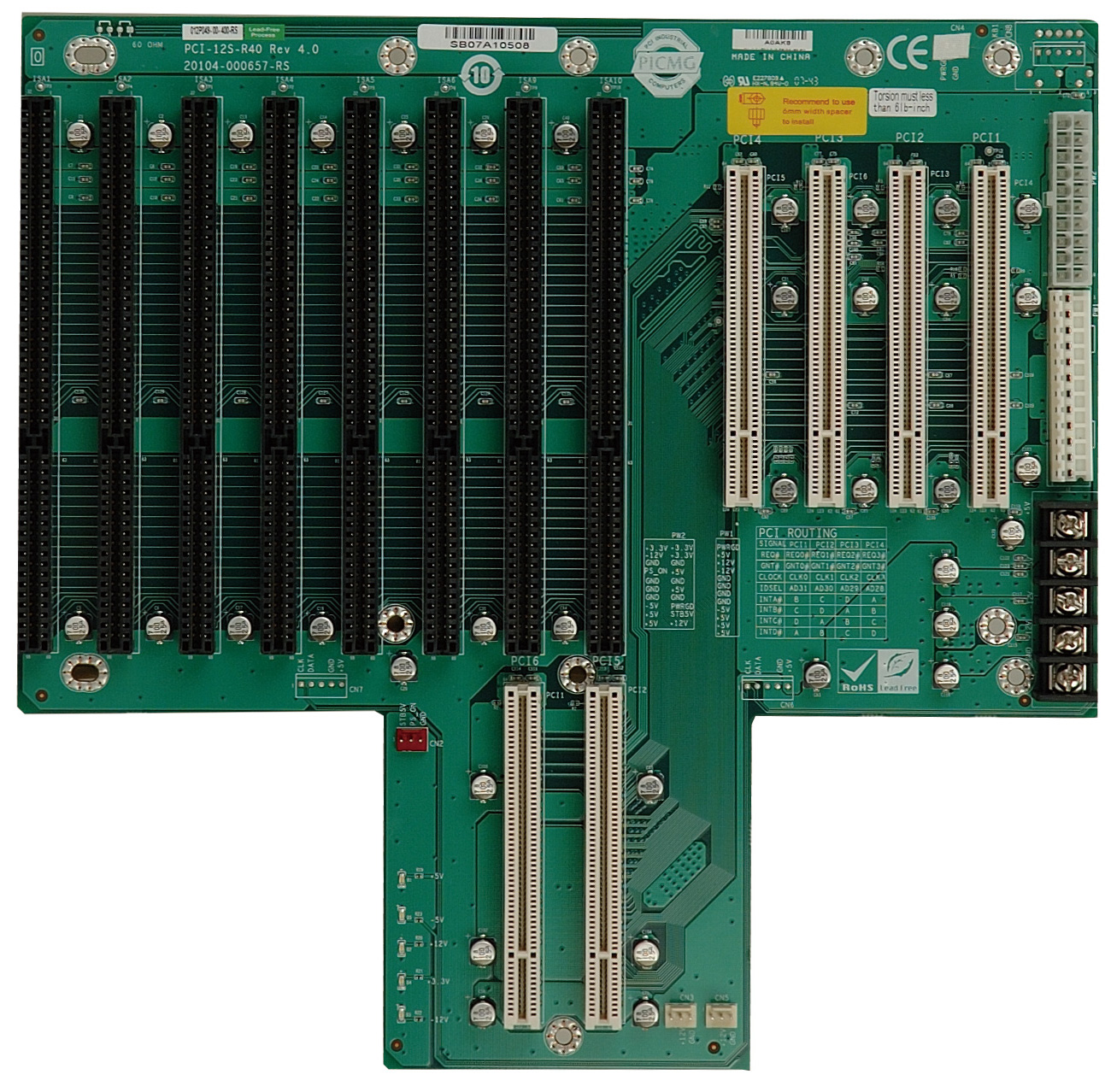
When we presented the virtualization layers earlier we didn’t mentioned GPUs as they are not available on every hardware platform. This issue had been identified a while ago by Nvidia when then decided to push their Nvidia GPU Cloud (NGC) platform. But adding a containerized technology on top will help you to hence reproducibility and flexibility while playing with different frameworks. This is why Virtualization is an awesome that allow you to break and rebuild you environment. Playing a bit with Deep Learning framework and with CUDA version ensure you a massive headache by the end of the day. Indeed a big part of the pain in AI when it comes to workload management is making sure every library is compiled with the right CUDA accelerators ( cuBLAS, cuDNN) associated to the right CUDA version with the right Driver. When running AI workload it’s often recommended to use Docker. Now… Let’s talk about AI and GPU’s Why AI practitioners loves virtualization and containerization The Different components can also be represented in form of a stack. – NEMU focuses on KVM support to better leverage the CPU’s virtualization extension and therefore reduce the need to translate instructions to the CPUs. – NEMU is a fork of QEMU that focuses on modern CPUs used with advanced virtualization features to increase the speed of existing QEMU implementation. – Supports all virtualizations/emulations. It’s basically converting input guest instruction (for instance ARM) into the actual hardware compatible instructions (for instance x86). – Emulates the processor and peripherals. Manages/Manipulates Virtualization (API, CLI and a Deamon – libvirtd) In oposition to QEMU, KVM leverage the Virtualization extension provided by the CPU itself without any emulation ( VT-x or AMD-V) All of these tools have specific roles as explained below : Tool
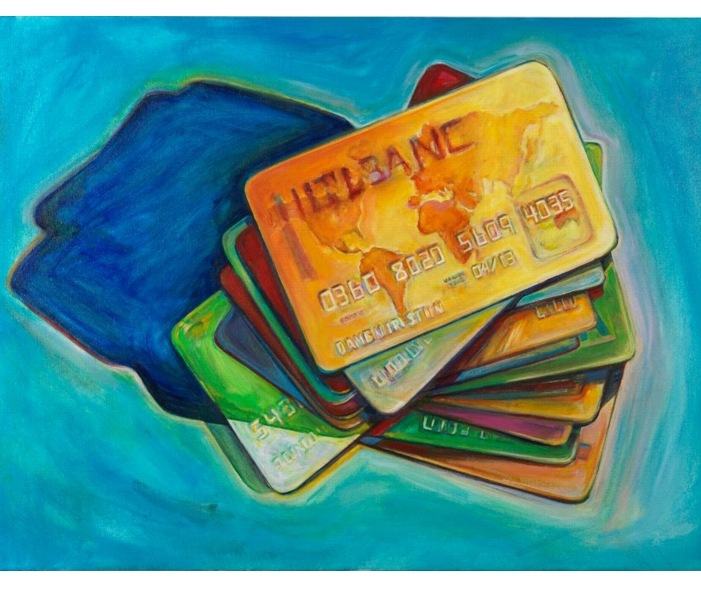
Some of you might know QEMU/NEMU/KVM/Lib Virt.
